Unlocking Business Potential with Data Labeling in Machine Learning
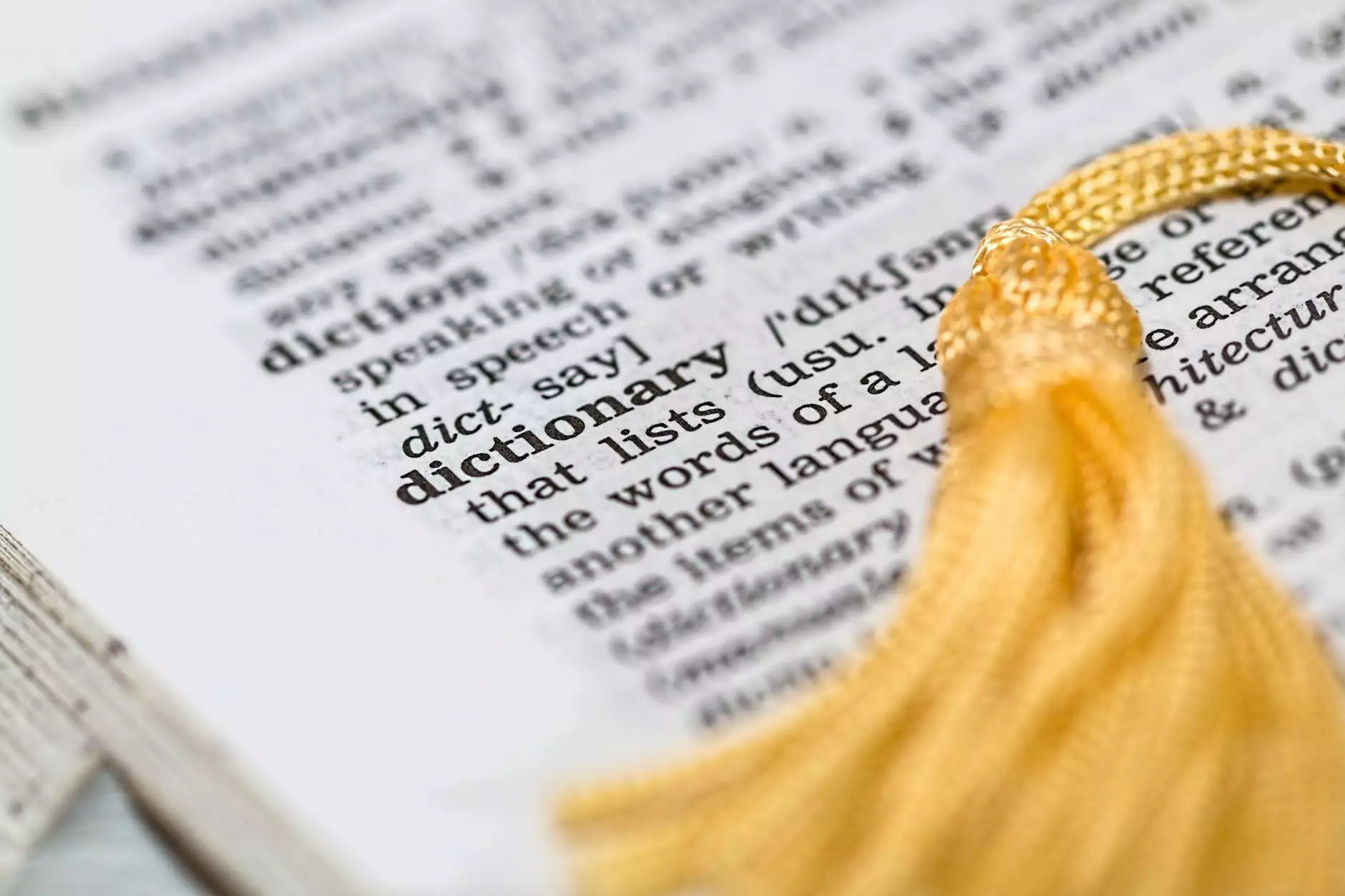
As the world becomes increasingly reliant on digital technologies, businesses across various sectors are recognizing the critical role of data in driving growth, efficiency, and innovation. Among the myriad of data-driven techniques, machine learning has emerged as a revolutionary force. However, to harness the full potential of machine learning, businesses must engage in effective data labeling. In this article, we will explore the intricacies of data labeling in machine learning, its significance for businesses, and how it can help you unlock new opportunities.
Understanding Data Labeling
At its core, data labeling is the process of annotating or tagging data with relevant information to train machine learning models. This involves the classification of data points so that algorithms can learn patterns and make predictions. For instance, in image recognition tasks, data labeling might include tagging images with the appropriate categories, such as "cat", "dog", or "car".
Why is Data Labeling Crucial for Machine Learning?
The efficacy of machine learning models heavily relies on quality data representation. Data labeling ensures that the data fed into machine learning algorithms is accurate, consistent, and representative of real-world scenarios. Here are several reasons why data labeling is vital:
- Improved Accuracy: Labeled data helps algorithms understand the context and intricacies of the data, leading to better performance and accurate predictions.
- Enhanced Learning: Proper labeling allows machine learning models to learn effectively from training data, refining their ability to generalize to unseen data.
- Model Training: Without labeled data, supervised learning, one of the most common machine learning paradigms, would be impossible.
- Reduced Bias: Comprehensive and diverse labeled datasets can help mitigate biases in machine learning models, leading to fairer outcomes.
How Businesses Benefit from Data Labeling
As businesses integrate machine learning into their operations, the importance of data labeling becomes ever clearer. Here are some key benefits that businesses can derive from effective data labeling:
1. Enhanced Customer Insights
With accurate data labeling, businesses can gain unprecedented insights into customer behavior and preferences. By analyzing labeled data, organizations can identify trends and make data-driven decisions that enhance customer satisfaction.
2. Streamlined Operations
Machine learning models can automate routine tasks, optimizing business processes. Properly labeled datasets are essential for training models that can, for example, manage inventory, forecast sales, and even automate customer service interactions.
3. Competitive Advantage
In an increasingly competitive marketplace, having access to high-quality labeled data can provide businesses with a significant edge. Organizations that leverage machine learning to analyze and respond to market trends promptly can outperform their competitors.
4. Innovation in Product Development
Data labeling allows businesses to fine-tune products based on customer feedback and usage patterns. By analyzing labeled datasets, organizations can innovate and develop products that better meet customer demands.
Best Practices for Data Labeling
To maximize the benefits of data labeling, businesses should consider the following best practices:
- Define Clear Guidelines: Establish comprehensive labeling guidelines that specify how data should be labeled to ensure consistency and accuracy.
- Utilize Quality Tools: Invest in high-quality data labeling tools and software that streamline the labeling process and improve accuracy.
- Leverage Human Expertise: While automated tools can assist, having domain experts review and label data can significantly enhance quality.
- Ensure Diversity in Data: Strive for diversity in the datasets used for training to promote fair and unbiased machine learning outcomes.
Challenges in Data Labeling
While data labeling is integral to machine learning, there are several challenges businesses may face:
1. Time-Consuming Process
Data labeling can be a labor-intensive task, especially when dealing with vast datasets. This can slow down the machine learning development cycle.
2. Subjectivity in Labeling
Different labelers may interpret and categorize data differently, leading to inconsistencies. This subjectivity can affect the quality of machine learning models.
3. Cost Implications
Hiring expertise for data labeling can incur significant costs. Businesses need to balance the expense against potential returns from improved machine learning performance.
Technological Solutions for Efficient Data Labeling
Fortunately, advancements in technology are paving the way for more efficient data labeling. Some innovative approaches include:
- Automated Annotation Tools: These tools use machine learning techniques to assist in the labeling process, reducing time and labor costs.
- Crowdsourcing Platforms: Businesses can utilize crowdsourcing to distribute labeling tasks across a large group of individuals, speeding up the process.
- Active Learning: This approach involves using a machine learning model to identify the most informative data points to label, optimizing the labeling effort.
- Labeling Services: Consider outsourcing data labeling to specialized companies that provide quality annotation services.
Key Industries Leveraging Data Labeling
Many industries are capitalizing on the benefits of data labeling in machine learning, including:
1. Healthcare
Data labeling is vital in healthcare for tasks such as diagnosing diseases from imaging data and predicting treatment outcomes based on patient data. Effective labeling can enhance healthcare outcomes and operational efficiency.
2. Automotive
In the automotive sector, data labeling supports the development of self-driving technologies. Annotating sensor data helps train autonomous vehicles to make safe and reliable decisions on the road.
3. Retail
Retailers are using data labeling to analyze consumer behavior and optimize inventory management. Labeled data enables better personalization in marketing efforts, enhancing customer engagement.
Conclusion
In conclusion, the process of data labeling in machine learning is not just a technical task; it is a crucial component that can determine the success of machine learning initiatives in any business. By recognizing its importance and implementing best practices, organizations can not only improve operational efficiencies but also gain a substantial competitive advantage. As we look to the future, businesses that embrace advanced data labeling methodologies will likely lead the charge in innovation and customer satisfaction.
For organizations committed to staying ahead in their industries, investing in effective data labeling strategies is essential. Whether you leverage internal resources, partner with external experts, or explore innovative technological solutions, the path to enhanced machine learning begins with quality data labeling.
As the landscape of business continues to evolve, one thing remains clear: data labeling will be a cornerstone of thriving enterprises that aim to innovate, connect, and succeed in an increasingly data-driven world.
data labeling machine learning