The Essential Role of Data Labeling in Machine Learning
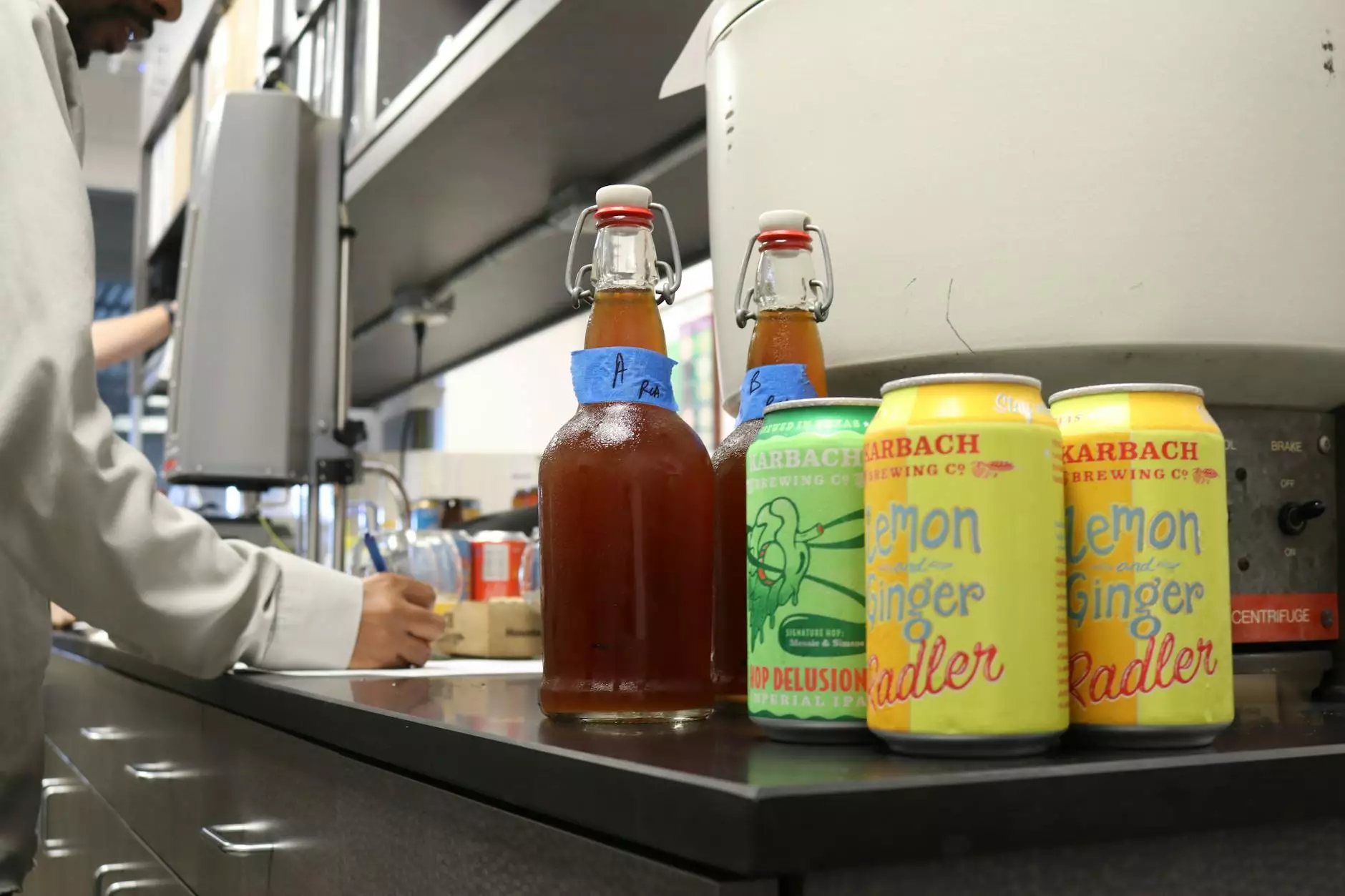
In today’s digital age, the importance of data cannot be overstated. As businesses increasingly adapt to technological advancements, the role of data labeling in machine learning becomes crucial. It's not just about collecting data; it’s about organizing and labeling it effectively to train machines that can think, learn, and make decisions. This article delves deep into how data labeling is transforming industries, particularly in the sector of Home Services and Keys & Locksmiths.
Understanding Data Labeling and Its Impact
Data labeling is the process of annotating data to make it understandable for machine learning algorithms. By assigning labels to data—such as images, text, or videos—businesses can train models to recognize patterns and make predictions. In the realm of machine learning, accurate data labeling is critical. Here’s why:
The Backbone of Machine Learning
Machine learning algorithms rely heavily on data to learn and improve over time. With properly labeled data, these algorithms can identify trends, conduct complex analyses, and provide insights that were previously unattainable. For businesses like KeyMakr, specialized in Home Services and Keys & Locksmiths, this means enhanced services and operational efficiency.
The Process of Data Labeling
Data labeling involves several critical steps:
- Data Collection: The first phase is gathering relevant data that needs labeling. This could include images of keys, textual descriptions of locksmith services, or customer reviews.
- Annotation: Once data is collected, the next step is annotating this data to provide context.
- Quality Assurance: Implement a rigorous quality control process to ensure that labels are accurate and reliable.
- Model Training: Use the labeled dataset to train machine learning models, allowing them to learn and make predictions.
Importance of Data Labeling in Business
For businesses in the Home Services and Locksmith sectors, effective data labeling provides numerous advantages. These include:
1. Enhanced AI Models
Properly labeled data allows for the development of sophisticated AI models. For instance, a machine learning model trained on labeled images of keys can facilitate key duplication or identification processes seamlessly.
2. Improved Customer Service
With machine learning models equipped with accurate data, businesses can provide personalized customer service. Imagine a locksmith service that uses AI to understand customer needs based on previous interactions and furnishes tailored recommendations!
3. Operational Efficiency
By harnessing machine learning, organizations can automate routine tasks, significantly enhancing operational efficiency. This leads to faster service delivery and reduced costs.
Data Labeling Techniques
There are various techniques and methods businesses can utilize for data labeling:
- Manual Labeling: Human annotators read and label data manually. Though time-consuming, it often ensures higher accuracy.
- Automated Labeling: Leveraging AI tools to label data automatically. This can speed up the process but may require subsequent quality checks.
- Crowdsourcing: Engaging a larger audience to label data, which can foster diversity in annotation but may compromise consistency.
The Challenges of Data Labeling
Despite its advantages, data labeling comes with challenges:
1. Scale
As businesses grow, the volume of data increases exponentially making it hard to label at scale without diminishing quality.
2. Subjectivity
Data labeling can sometimes be subjective. Different annotators might interpret the same data differently leading to inconsistencies.
3. Training Costs
Training machine learning models requires significant investment in time and resources, which might deter smaller businesses.
Case Studies: Data Labeling in Action
Numerous companies have harnessed effective data labeling to enhance their services:
KeyMakr: A Modern Locksmith Solution
Take KeyMakr as an example. By incorporating data labeling into their service model, they have successfully trained AI to identify and replicate various types of keys. This innovation not only improves operational efficiency but also provides unmatched customer service. By analyzing customer feedback through labeled data, KeyMakr is better positioned to understand service trends, customer preferences, and areas for improvement.
Home Security Firms
Home security firms are leveraging data labeling to enhance their surveillance systems. By labeling footage accurately, these companies can train their AI to recognize unusual activities, thus providing homeowners with better security solutions.
Future Trends in Data Labeling
As the landscape of machine learning evolves, so does data labeling:
- Increased Use of Automation: More automated solutions will emerge, making labeling faster while still maintaining accuracy.
- Integration with AI: Future machine learning models will likely require fewer labeled examples, thanks to advancements in transfer learning and unsupervised learning techniques.
- Real-time Annotation: As technology grows, businesses will be able to label data in real time, facilitating quicker responses and actions.
Conclusion
Data labeling is a vital step in the machine learning process that shapes the future of various industries, including Home Services and Keys & Locksmiths. By transforming raw data into structured information, businesses can make data-driven decisions and craft personalized customer experiences. As companies like KeyMakr adopt machine learning powered by data labeling, they stand to gain a competitive advantage in a technology-driven market.
In summary, leveraging the power of data labeling in machine learning will enable businesses to maximize their operational efficiency, improve their offerings, and adapt to the ever-evolving technological landscape.
data labeling machine learning